Precision in sight: How AI-powered visual inspection improves industrial quality control
Focusing on product quality is essential for manufacturers and service providers in today’s competitive scenario.
Poor quality control can lead to significant operational and financial losses, including wasted raw materials, decreased sales, and increased costs associated with recalls and repairs.
Traditional manual visual quality inspection methods are still widely used across various industries. However, these methods have limitations, such as inefficiency, high manpower and training costs, and the need to comply with stringent safety and working hours regulations.
Artificial Intelligence (AI) offers a more effective solution for quality control. AI-based visual inspection uses advanced machine vision algorithms to detect quality issues accurately. This technology minimizes errors, optimizes resource use, and ensures superior product quality. This article discusses the concept of AI-based inspection in product quality inspection, its advantages, and its implementation.
Concept of AI-based visual inspection
The AI-based quality Inspection process in an industrial setting involves steps designed to detect defects and ensure product quality. The following is a breakdown of how an AI system operates, from image acquisition to final decision-making and documentation:
- Image capturing: The process begins with the precise acquisition of images, where high-resolution cameras or sensors capture high-resolution images or videos of the product along the product line. These image-capturing devices continuously capture detailed images or 2D/3D scans of the product from various angles as they move along the line.
- Image enhancement: Captured images may need enhancement to improve clarity and highlight relevant features. This step reduces distortions or irrelevant details, enabling the AI systems to analyze and decode the information more accurately.
- Feature extraction: The processed images are then analyzed by AI-based effect algorithms, which analyze critical aspects such as color depth, patterns, shapes, and textures. This analysis transforms raw image data into a format suitable for input to the machine learning algorithm.
- Defect detection: The processed images are then analyzed by an AI-based defect detection system. Trained models compare the extracted features of the images from the already-known patterns of defects. This helps in effective quality control by identifying defective and non-defective products.
- Report generation: After inspection, the quality control team generates a detailed report of the inspection results. The report includes information on detected defects and their locations.

Figure 1: Steps involved in AI-based visual inspection
Benefits of AI-based visual inspection
AI-based visual inspection offers the following advantages in the quality control process:
- Increased accuracy and consistency: AI-based visual inspection systems are not prone to fatigue, distractions, or biases that can affect human inspectors, leading to more consistent and accurate defect detection. They can also detect the smallest defects with high precision, ensuring that only high-quality products pass inspection.
- Enhanced speed and efficiency: AI-based systems can analyze images and data in real-time, allowing for immediate identification and correction of defects without slowing down production lines. These systems can operate continuously without breaks, maximizing throughput and operational efficiency.
- Cost reduction: AI automates the inspection process, reducing the need for manual labor, and significantly lowering operational costs. Accurate defect detection also reduces the rework needed and minimizes the production of defective products, thereby reducing material waste and associated costs.
- Scalability and flexibility: AI-based inspection systems are highly adaptable because they can be easily adapted to inspect different types of products and detect various defects. They can be scaled depending on the production volume, making them suitable for small-scale and large-scale operations.
- Improved product quality: With AI implementation, manufacturers can enforce stricter standards, improving product quality and customer satisfaction. AI systems can continuously learn from new data, improving accuracy and adapting to new defect types.
- Data-driven insights: AI systems generate a wealth of data that can be analyzed to identify trends, optimize production processes, and predict potential issues before they occur. By analyzing defect patterns, AI can help predict when machinery will likely fail, enabling proactive maintenance and reducing downtime.
- Environmental benefits: AI inspection systems reduce material waste by minimizing the production of defective products, leading to more sustainable manufacturing practices. With fewer defects and less rework, production processes become more energy-efficient, contributing to overall environmental sustainability.
Omron’s FH series AI-based defect detection system
The FH Series Vision system by Omron is an AI-based defect detection system that does not require learning samples. The system mimics the techniques skilled inspectors use, thanks to its AI-based image filter that can accurately identify defects on any product background.

Figure 2: Omron’s FH series AI-based automated visual inspection system Configuration
Figure 2 shows the FH Series Vision AI-based defect detection system with different components. The system includes high-resolution cameras that capture high-resolution images suitable for sensory inspection at high speeds. These cameras use Multi-Trigger Imaging, enabling them to capture images and process them simultaneously, leveraging the speed of the multi-core processor to capture long objects at high speeds. The processed images are sent further to the FH series camera controller, where they are analyzed for defects using an AI-based algorithm. It also stores the inspection image data required for defect-cause analysis. The FH Series can assign a unique ID to each inspection image and its result, which are then output to the host device. This feature allows for quick retrieval of inspection images and helps efficiently identify the causes of failures, as depicted in Figure 3.
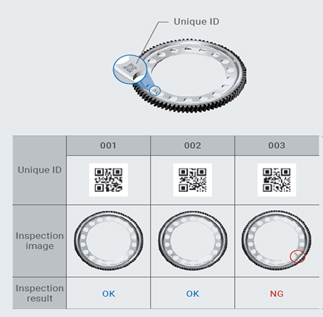
Figure 3: Defect analysis of products using Omron’s FH series AI-based defect detection system
The FH series controller uses Sysmac Studio software (an integrated development environment), which enables simulation and tracing based on information acquired from the vision system. It is also used to configure, program, debug, and maintain machine automation controllers, including OMRON’s NJ/NX-series CPU Units, NY-series Industrial PCs, EtherCAT Slave Units, and HMIs.
Stay informed
Keep up to date on the latest information and exclusive offers!
Subscribe now
Thanks for subscribing
Well done! You are now part of an elite group who receive the latest info on products, technologies and applications straight to your inbox.
Read more on:
Artificial Intelligence: AI at the Edge - element14 Community
Related Articles
- Advanced ML for MEMS Sensors: Enhancing the Accuracy, Performance, and Power consumption
- How to design a MEMS Vibration Sensor for predictive maintenance
- Precision in Sight: How AI-powered visual inspection improves industrial quality control
- How to Capture High-Quality, Undistorted Images for Industrial Machine Vision
- Robotics and AI Integration: Transforming Industrial Automation
- The modern challenges of facial recognition
- Intelligent cameras for smart security and elevated surveillance
- Evolution of voice, speech, and sound recognition
- AI and IoT: The future of intelligent transportation systems
- Machine Vision Sensors: How Machines View the World
- Demystifying AI and ML with embedded devices
- How to implement convolutional neural network on STM32 and Arduino
- How to do image classification using ADI MAX78000
- The Benefits of Using Sensors and AI in HVAC Systems
- Deep learning and neural networks
- Latest Trends in Artificial Intelligence
- Hello world for Machine Learning
- How to implement AR in process control application